Managing research data
Philippa Frame
Getting started
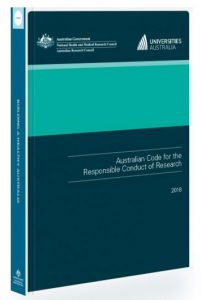
introduction to research data management
Researchers have a responsibility to ensure their data is accurate, complete, authentic and reliable. Good research data management practices ensure that researchers and institutions can meet their obligations to funders, improve the efficiency of research, and make data available for sharing, validation and reuse.
Publication and re-usability of research data bring great benefits such as reproducibility and transparency, enhanced reputations of researchers and institutions, funder agreement compliance, and compliance with Open Access agendas. To support these objectives, it is imperative that research data management is done properly from the outset, through all stages of the research data lifecycle.
In Australia, all researchers are required to comply with the Australian Code for the Responsible Conduct of Research, 2018 (the Code) which outlines the responsibility researchers have to ensure the safe and secure storage and management of research data, records and primary materials, and where possible and appropriate, to allow access and reference to these data.
Adherence to the guidance set out within the QUT Manual of Policies and Procedures (MoPP) D/2.8 Management of research data and primary materials will allow QUT researchers to comply with the Code.
what is research data?
According to the MoPP D/2.8, research data is :
- data in the form of facts, observations, images, computer program results, recordings, measurements or experiences on which an argument, theory, test or hypothesis, or other research output is based.
- It relates to data generated, collected, or used, during research projects, and in some cases may include the research output itself.
- Data may be numerical, descriptive, visual or tactile.
- It may be raw, cleaned or processed, and may be held in any format or media.
- Research data, in many disciplines, may by necessity include the software, algorithm, model and/or parameters, used to arrive at the research outcome, in addition to the raw data that the software, algorithm or model is applied to.
types of data
Depending on the kind of research or method of analysis, different types of research data may be created or collected. These types include:
Observational data are captured in real time, usually unique and irreplaceable.
e.g. brain images, survey data.
Experimental data are from lab equipment, often reproducible, but can be expensive.
e.g. chromatograms, microassays.
Simulation or model data are generated from test models where model and metadata may be more important than output data from the model.
e.g. economic or climate models.
Derived or compiled data result from processing or combining ‘raw’ data, often reproducible but expensive.
e.g. compiled databases, text mining.
Reference or canonical data are a (static or organic) conglomeration or collection of smaller (peer reviewed) datasets, most probably published and curated.
e.g. gene databanks, crystallographic databases.
why manage research data?
Research data, and the primary materials from which they are derived, are valued assets and outputs for researchers as well as the institutions with which they are affiliated.
They must be properly managed to enable:
- verification of findings
- reproducibility and transparency
- protection against loss
- protection of sensitive or confidential information
- data reuse (for yourself and others)
- compliance with codes of conduct
- statutory retention, funding body, publisher and QUT policy compliance.
Other benefits of managing your data include:
- Increased research efficiency, saving time and resources.
- Facilitating future research by allowing others to build on or add to your research data.
- Increased citations of research data and of publications based on that data.
Planning for how data will be managed throughout a research project ensures these benefits are realised. Data management planning usually follows a research data lifecycle approach.
the research data lifecycle
The term ‘research data lifecycle’ refers to the various stages within the lifespan of data, from the point of creation or collection through to dissemination, and will usually continue after the research project that creates it has concluded.
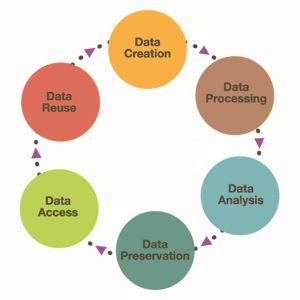
As part of the process of producing your data management plan, you will need to think about your research and the data that you produce as part of a lifecycle: from creating the data, to documenting and analysing it, organising and storing it, preserving and sharing it, and ultimately to its potential re-use in further research.
There are many different iterations of the research data lifecycle that exist, but generally, they all follow a similar cycle to the one developed by NTU Singapore.
Data may have a longer lifespan than that of an MPhil or PhD or a 5-year funded project. For example, a researcher might continue to work on aspects of their data after they’ve completed their thesis; follow-up projects might arise in which they re-analyse their data or combine it with other data. Their data might also be re-used by other researchers who pursue related research projects long after their thesis has been accepted. Well organised, well documented, and properly preserved data is easy to access, review and share; as such, it is invaluable to the advancement of knowledge.
Pennock (2007) highlighted in Digital Curation: A Life-Cycle Approach to Managing and Preserving Usable Digital Information that the lifecycle approach is necessary because:
- Digital materials are fragile and susceptible to change from technological advances throughout their lifecycle, i.e. from creation onwards;
- Activities (or lack of) at each stage in the lifecycle directly influence our ability to manage and preserve digital materials in subsequent stages;
- Reliable re-use of digital materials is only possible if materials are curated in such a way that their authenticity and integrity are retained.
Exercise: Find a research data life-cycle model from another institution. How similar or different is it to the above?
data management planning
Data management planning is an internationally accepted practice that provides a recognised structure for the organisation and documentation of research data.
Effective research data management also plays a vital role in managing research risk. All re search is subject to a range of data-related risks such as data loss or corruption, and privacy or copyright breaches. These risks come with significant, potentially catastrophic impacts. Effective research data management can go a long way towards preventing and managing such risks.
At QUT, researchers can use the Data Management Planning Tool to consider their data management strategy and document it in a data management plan.
Each field provides links to related policies, agreements, responsibilities and resources.
The tool allows researchers to:
- reflect on how you’ll handle your data during and after your research and helps you identify and clarify your specific research data needs
- engage with various aspects of research data management such as relevant policies and guidelines, resources, services and contacts.
- ensure your plan will allow your work to meet the highest standards of integrity, so that your data is accurate, complete, authentic and reliable
- communicate or share your plan with your supervisor or as supplementary documentation for grant applications
- fulfill your responsibilities as a QUT staff member, PhD student or MPhil student.
All QUT researchers must complete a data management plan for all research projects for which they are responsible. With some exceptions, PhD students are required to complete a data management plan before submission as part of their Stage 2 milestone, and MPhil students are required to consider completing a data management plan before submission as part of their Research Proposal milestone.
Learn more
The resources listed below can give you a greater understanding of how to manage research data.
- QUT’s IFN001: AIRS Module 8 Managing data provides information from a higher research degree student’s perspective
- Australian National Data Service (ANDS)
- Australian Research Data Commons (ARDC) (formerly ANDS)
- ANDS Guide: Research Data Management in Practice
- DCC Curation Lifecycle Model
- UK Data Service
Consider: Look at the data management plans below. How do they compare to data management plans at your institution?
Australian:
- Data management plans and toolkits for most Australian universities (see Data Management Tools Column)
- ANDS Guide: Data Management Plans
International:
- Using the FAIR Principles in DMPs from European Union Horizon 2020
- DMPonline from Digital Curation Centre UK
- DMPTool – public Data Management Plans from the US
- Data Management Planning – from DataOne
- Framework for Research Data Management Makes Life Simpler for Researchers – Science Europe Working Group on Research Data
Discipline-specific:
- National Science Foundation USA offers a range of DMP examples by research area
- Managing research data in Archaeology from the Archaeology Data Service in the UK
- Australian Antarctic Data Centre Data Management Plan
- Example DMP for a Biology study from Curtin University
- Example UK DMPs – from many disciplines
- Example Coastal and Marine Science DMP – from the USA
- Example Humanities DMPs – from National Endowment for the Humanities USA
- Sample DMP for Biosciences – from American University USA
Challenge me
Attribution
Content in this chapter has been developed by QUT Library.
All information correct at time of publication, 8 October 2021.
references
D/2.8 Management of research data and primary materials – QUT Manual of Policies and Procedures
MANTRA – University of Edinburgh online research data management training
ANDS – Australian National Data Service
image credits
Australian Code for the Responsible Conduct of Research, 2018 – National Health and Medical Research Council
Research Data Lifecycle – Nanyang Technological University, Singapore